The RHODAS project is dedicated to advancing the performance, control, and health of Integrated Motor Drive (IMD) systems within heavy-duty electric trucks. Central to this initiative is the development of a sophisticated Decision Support System (DSS) designed to enhance fault detection, diagnosis, and management.
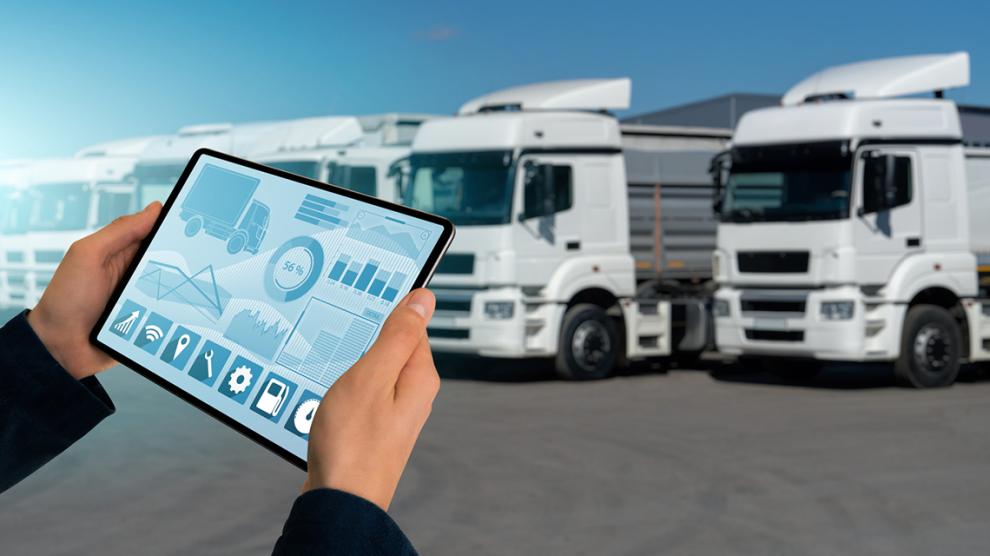
This system collects and analyses fault data from various truck components, such as the powertrain and gateway, providing real-time insights and actionable recommendations to operators and engineers. By leveraging advanced AI and Machine Learning (ML) models and rule-based methods, the Decision Support System aims to optimise the reliability and efficiency of electric trucks, ensuring they meet the evolving needs of Original Equipment Manufacturers (OEMs) and end-users.
The DSS framework is built on a robust backend strategy that includes data preparation, fault classification, and fault management. Data from the truck's monitoring tools are processed to detect anomalies and classify potential faults. The system prioritises these faults based on severity, ensuring that critical issues are addressed promptly. Additionally, the DSS accumulates historical fault data, contributing to a comprehensive knowledge base that OEMs can use to improve future powertrain designs. This strategic approach not only enhances real-time fault management but also supports long-term operational planning and continuous improvement.
A key feature of the DSS is its integration with a Knowledge Graph, which provides a structured and interconnected representation of the truck's components and their relationships. This visual and semantic model enables more effective analysis and decision-making, helping operators understand complex fault interactions and predict potential failures. The Knowledge Graph is constructed using Failure Mode and Effects Analysis (FMEA) data, which systematically identifies, prioritises, and mitigates potential failures. By incorporating FMEA into the Knowledge Graph, the DSS offers a powerful tool for fault diagnosis and preventive maintenance, improving the overall reliability and operational efficiency of the electric truck.
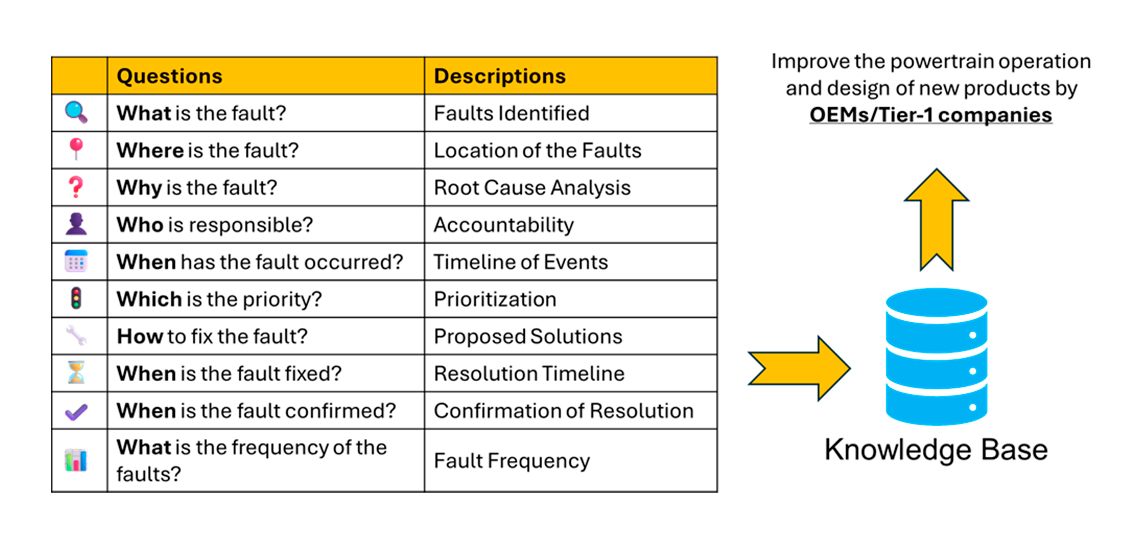
The project also emphasises the importance of predictive maintenance, utilising smart non-invasive sensors to monitor the long-term performance of the powertrain. These sensors, including current, ultrasound and vibration detectors, provide early indicators of potential faults, allowing for proactive maintenance strategies. Data collected from these sensors are used to develop AI-based or rule-based models for fault detection and prediction at the gateway level. This approach ensures that maintenance interventions can be scheduled before functional failures occur, minimising unplanned downtimes and repair costs.
The RHODAS DSS is designed with a user-friendly interface that facilitates real-time decision-making. The dashboard provides a comprehensive view of fault statuses, including their history, root causes, and recommended actions. Users can interact with the system to validate faults, track resolutions, and understand system risks through the interactive Knowledge Graph. This intuitive design ensures that users can easily access and interpret critical data, enhancing their ability to make informed decisions.
In conclusion, the RHODAS project represents a significant advancement in the field of electric truck technology. By integrating advanced fault detection, diagnosis, and management tools, the DSS enhances the performance and reliability of IMD systems. The project's commitment to continuous improvement and proactive maintenance strategies sets a new standard for the industry, ensuring that electric trucks can meet the demands of a rapidly evolving market. Through collaboration and innovation, RHODAS is paving the way for a more resilient and efficient future in heavy-duty electric transportation.